Data analysis learning: Know your universal set. In our case your user segments.
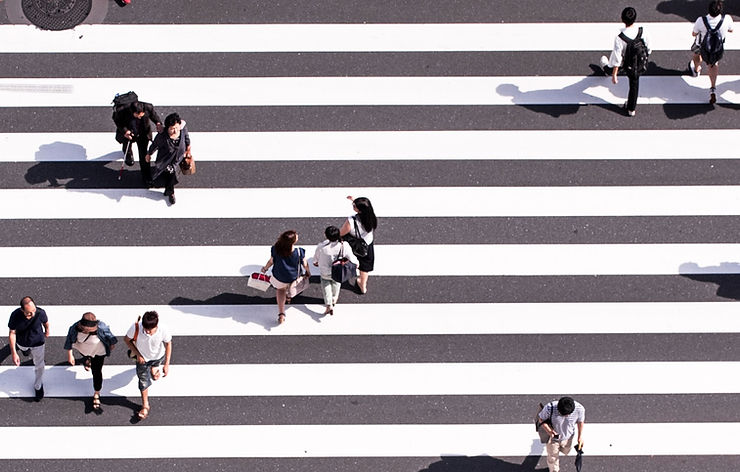
Background
Recently I mentioned on LinkedIn that I had unsubscribed from Netflix because they keep killing series after season 1 or 2 with a cliffhanger, and I don’t want more cliffhangers sitting in my head. Here’s the original post.
Netflix is known for making decisions based on numbers. I don’t want to argue on their numbers, obviously I don’t know their numbers. But some arguments under my post made me decide I want to make aware on the bias that sneaks in when we forget to closely look at our universal set.
What is “the universal set”
“Universal Set Definition. Universal Set is defined as a set that contains all the elements or objects of other sets, including its own elements.” – Collegedunia
In German it’s called “Die Grundmenge” or “Die Grundgesamtheit”. I had to look up the correct statistical term in English for this article. I used to call it “base set”.
When we want to analyse product usage, our universal set contains multiple different user segments. Note that there can be other ways of looking at a universal set but for our needs as non-statisticians who want to get an understanding of product usage I want to emphasize on sets as customer segments.
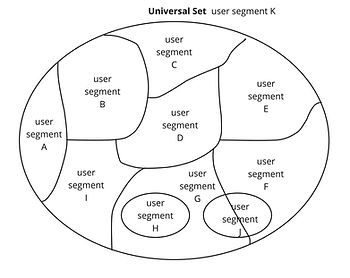
When I say “Know your universal set”, I mean understand that there are different segments that use your product, understand the dynamics between them and their differences, and understand the effect of their characteristics on the data you collect.
Let’s have a look at three considerations.
1. Who is your universal set, what do their signals mean, how do we need to serve them?
When you read these symptoms what do you think I’m describing:
- Loss of appetite
- Rash
- Diarrhea
- Increased temperature or fever
- Floppiness
- Irritability
- Insomnia
Are you thinking of an adult who has gastroenteritis?
These are potential symptoms of a teething baby!
Imagine you’d treat the baby like the adult… Fatal!
Context is important. The same information in a different context can lead to different insights. Therefore it’s important to know your universal set.
Applying this to Netflix:
In the Netflix case, when they analyse success of a series, what is the context of these numbers? When did the series run (seasonal effects), in which category did they compete (right industry), which series did it compete against (right competition), is there certain watching behavior depending on the type of series (e.g. the more flat the story, the more binge watching potential or actually the other way around?), but also what does the general market trend say (e.g. is binge watching still a thing), etc.
2. How does a shift in the universal set affect your numbers?
Imagine you run a restaurant. You serve your guests delicious French cuisine. Your guests are so amazed by the food and service, you get 5 stars across the board! You decide to experiment with fusion kitchen. Slowly you start getting bad reviews from your old guests, but at the same time you receive good reviews from your new customers (i.e. your new customer profile). If you look at the number of ratings themselves and average rating maybe nothing changes. But looking at the reviews and feedback regarding what your guests like and want to have more of, you see big differences. It’s your decision now who you want to serve: your former type of guests who want more French cuisine, or the new type of guest who want more fusion?
Depending on who you want to serve, your offering will change: You’ll either go back to more French cuisine or you’ll turn your menu into a fusion experience.
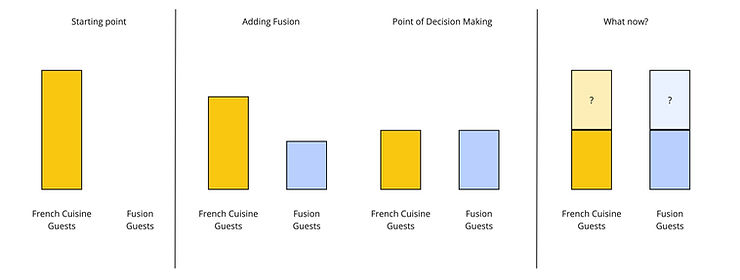
Now it becomes a strategic decision and a plus/minus game. Which market do you want to serve? What do trends and signals in your research tell you what to bet on? What’s the best mix of food to offer? Which KPIs are you optimizing for? Etc.
Applying this to Netflix:
In Netflix’s case they might optimize for short-term margin and want to find those series that are cheap in production and create binge watchers. Maybe they don’t care much about retention at the moment (which would be fatal considering that there are more and more streaming services out there)? Maybe they want to strategically change their customer group and therefore create an offering that is more suited to the new target group that they prefer?
Who knows 🤷🏻♀️
3. Mixing universal sets in data analysis
Imagine you work for a supermarket and need to make a decision: should you expand the offer for clementines or rather tangerines. The difference: Clementines have no seeds and a gentle flavor. Tangerines have seeds and an intense flavor. And very important market information: Generally, fewer and fewer shops offer tangerines because fewer and fewer farmers are growing them.
You analyse past purchase data and notice that more clementines were sold, relatively compared to the amount of clementines and tangerines offered. Boom you make your decision: expand clementines, reduce tangerines because clementines sold better in the past and overall sales have been stable for a while.
What you’ve not considered is that the offers from the past were not comparable. So far you offer organic clementines, premium not organic clementines, cheap not organic clementines vs. premium not organic and cheap not organic tangerines. What if number of clementines sold was higher because all organic buyers bought clementines only because there were no organic tangerines, although they’d preferred tangerines? What if this one buyer group made the difference? The data becomes incomparable because the sales numbers of the offers come from different buyer groups, which makes your universal sets incomparable.
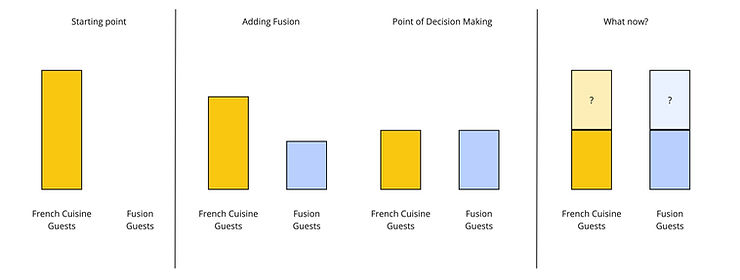
This is of course very much simplified. Ideally you have a strategic reason for your decision. But it’s often the case that you have to make decisions without clear strategic guidance.
Variation: Experiment
What if you ran an experiment instead of analysing past behavior, and in your experiment you’d have incomparable offers? Making decisions would become more risky because the data might misguide you?
Yes and no.
It might misguide you if your experiment setup was wrong and didn’t consider potentially incomparable target groups. What I mean is: For making numbers really comparable, you’d need to make only one difference in the variations. You’d either need to add organic tangerines to your test or remove the organic clementines, and make sure that prices of the three (or two) types of clementines and tangerines are the same for each type. If not, you’d need additional qualitative data to understand the different buying behavior.
Variation: Unpleasant aftereffects
Let’s continue with the decision to expand clementines and limit tangerines. If you’re a big supermarket with little competition, chances are high that nothing is going to change for you. People have to live with what you offer them.
If you are in a highly competitive market, however, the situation might become tricky.
What if another supermarket opens nearby which offers organic tangerines and clementines as well as not organic tangerines and clementines? You not only notice that sales of clementines drop but also sales of other goods. After some research you find out that your customers who mainly buy organic food started to shop in the other supermarket. You notice that the other supermarket doesn’t have a noticeably different organic food offering so you start interviewing the customers. Turns out they do the groceries over there because they offer something that has become very rare: Organic tangerines! Why go to multiple stores if this one store offers a specific type of organic food that is otherwise difficult to find?
Now was it a good decision or a bad decision to reduce tangerines and go full in on clementines?
It depends on many other considerations.
Even if sales of other goods dropped, was it overall a big revenue drop? Have other new offerings made up for the loss? How many organic food buyers have you lost? Is it strategically ok to lose them? Will they come back for other reasons? Etc…
Applying this to Netflix:
Let’s say I’m the organic food buyer type of series watcher. I’m willing to pay a higher price for a certain treatment of the food because I personally connect this with higher quality, and high quality food is important for me. The Netflix analogy could be that I’m willing to pay for the highest priced tier as long as I can watch series and movies that have a certain depth, a well thought through storyline, and sophisticated scenery or CGI that doesn’t look cheap, because I personally connect this with higher quality, and watching high quality content is important for me. Let’s say this is my definition of “high quality content”.
When Netflix kills one high quality series after a cliffhanger, this is not a tragedy. I might be disappointed because I want to know how it continues but there are enough other high quality series.
Netflix kills the next high quality series after a cliffhanger. Enough others. No problem.
Netflix kills one after the other. I read that they might have issues with series that have high production costs. More and more shallow and cheap series come out and stay for multiple seasons while deep and sophisticated series get killed after season 1 or 2.
What does this trigger in me?
In my case nothing good.
I could either continue or cancel my Netflix subscription.
Now let’s say all of us who are the same type of series watcher decide to cancel our subscriptions. At the same time more and more of the “easy watchers” (whatever that means but I know that I’m not easy 😄) start their subscription. The type of content that easy watchers like is different from what complicated watchers (like me) prefer.
What’s the effect on Netflix’s content?
Let’s say Netflix notices that series with a shallow story get better watch-points than complex series (I’m making “watch points” up to talk about some way how Netflix measures success of their series, how ever they do it). They analyse their segments and notice the shift. Like other companies they try to maximize ROI and therefore they need to find the series that optimize for their measure of ROI. I don’t know how they measure “return” but part of “investment” are for sure production costs. Like any other good decision maker, I would also think thrice if I wanted to continue a product (aka series) that creates a lot more costs than other series.
Now let’s keep these points in mind when we look at the following scenarios:
Scenario 1: Overall they gain more users than they loose. They decide to continue killing all series that have high production costs. Turns out, only the shallow series and some complex series survive this minimum cost approach.
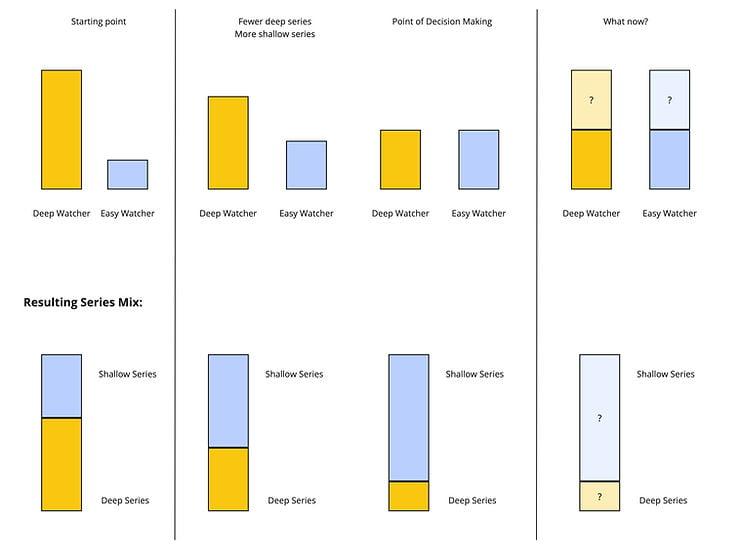
Scenario 2: They keep losing subscribers in a way that it hurts. They could now either follow the watch-points and the minimum cost approach and double down on converting easy watchers to Netflix subscribers which comes with a heavy marketing campaign. Or they could keep some of the deep but costly series to please complicated watchers so that they retain. This way they could stop the bleeding but would end up having higher production costs.
Are we ready to jump on the conclusion that they should invest into retaining their subscribers? I tend to say that, too.
HOWEVER – We are just gawkers.
It’s easy to make conclusions from outside. We judge “Why don’t they do this or that” or “What a stupid decision they made”. But we don’t know what’s happening inside. We don’t know what they truly take into consideration when they make decisions. We know what they share with us but we don’t know what we don’t know.
Conclusion
Data can be simple, but data can also be difficult. Only looking at data itself without knowing the context when where how and from whom it was collected from can lead you to wrong conclusions. Sometimes this doesn’t have any negative effect. And sometimes this can lead to product failure.
Therefore, understand the context of your data before jumping into conclusions.